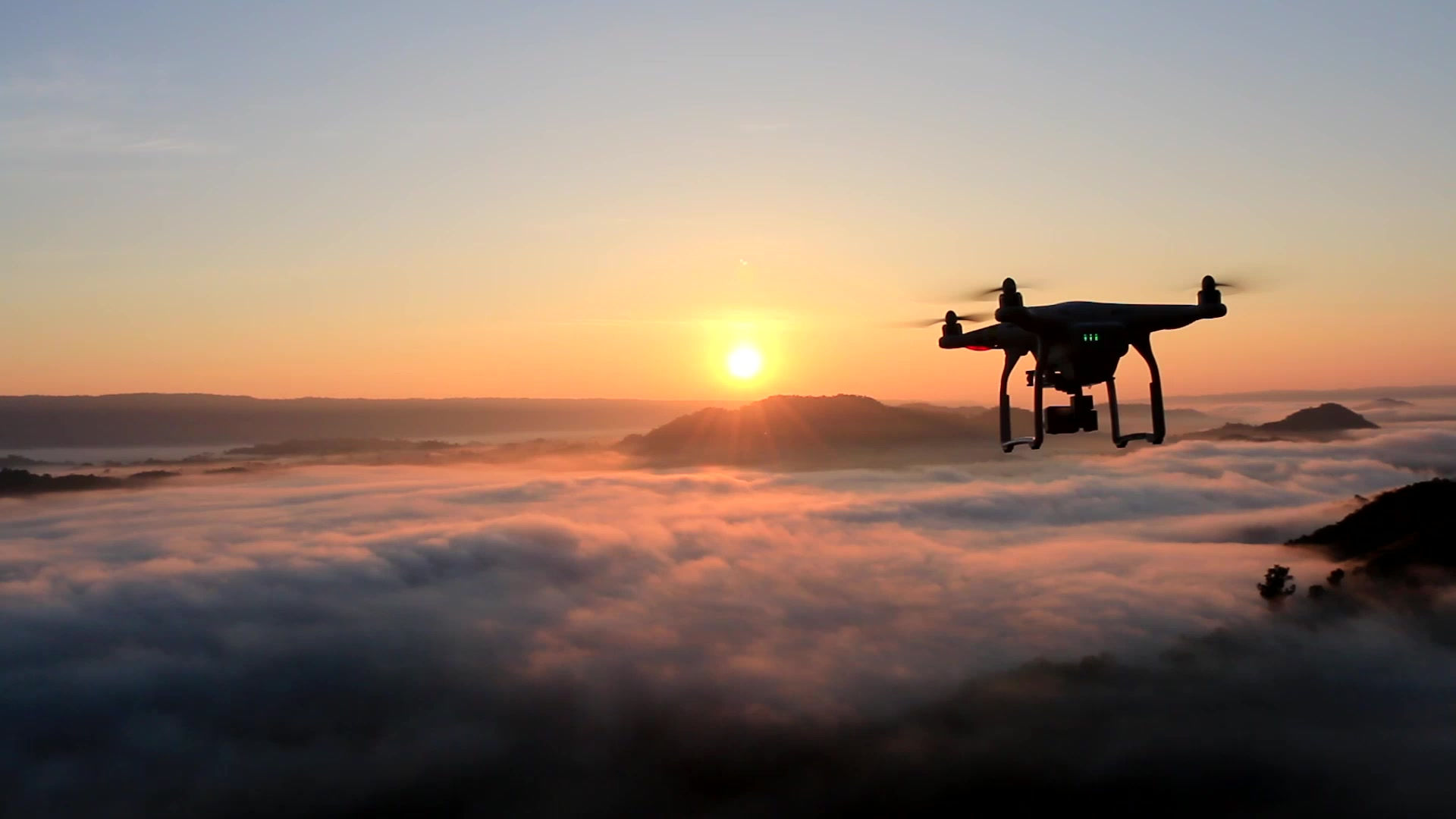
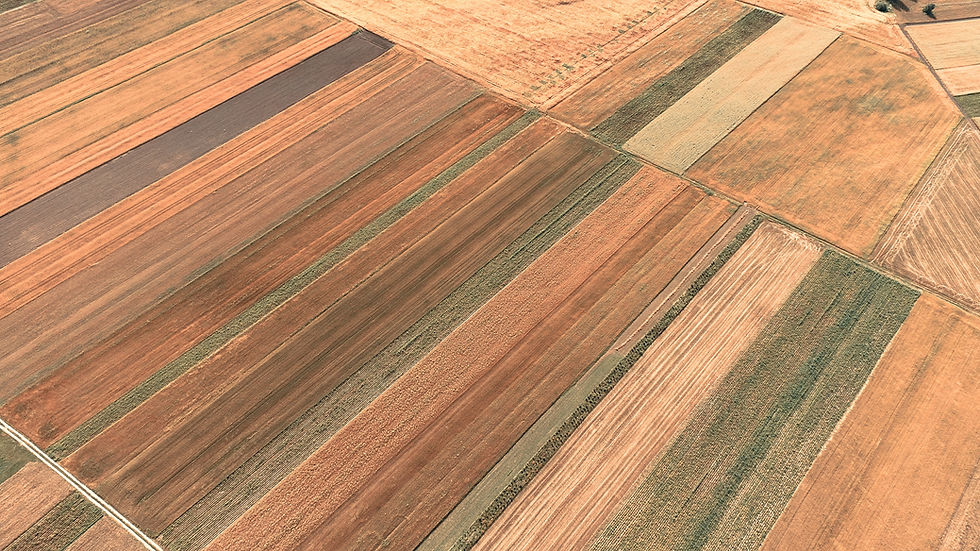
The Potential Of UAS Imagery For Soil Mapping At The Agricultural Plot Scale
Abstract: Soil mapping is expensive and time consuming. Airborne and satellite remote sensing data have already been used to predict some soil properties but now Unmanned Aerial Systems (UAS) allow to do many images acquisitions in various field conditions in favour of developing methods for better prediction models construction. This study propose an operational method for spatial prediction of soil properties (organic carbon, clay) at the scale of the agricultural plot by using UAS imagery. An agricultural plot of 28 ha, located in the western region of Paris France, was studied from March to May 2016. An area of 3.6 ha was delimited within the plot and a total of 16 flights were completed. The UAS platforms used were the eBee fixed wing provided by Sensefly® flying at an altitude from 60m to 130m and the iris+ 3DR® Quadcopter (from 30m to 100m). Two multispectral visible near-infrared cameras were used: the AirInov® MultiSPEC 4C® and the Micasense® RedEdge®. 42 ground control points (GCP) were sampled within the 3.6 ha plot. A centimetric Trimble Geo 7x DGPS was used to determine precise GCP positions. On each GCP the soil horizons were described and the top soil were sampled for standard physico-chemical analysis. Ground spectral measurements with a Spectral Evolution® SR-3500 spectroradiometer were made synchronously with the drone flights. 22 additional GCP were placed around the 3.6 ha area in order to realize a precise georeferencing. The multispectral mosaics were calculated using the Agisoft Photoscan® software and all mapping processings were done with the ESRI ArcGIS® 10.3 software. The soil properties were estimated by partial least squares regression (PLSR) between the laboratory analyses and the multispectral information of the UAS images, with the PLS package of the R software. The objective was to establish a model that would achieve an acceptable prediction quality using minimum number of points. For this, we tested 5 models with a decreasing number of calibration points: 20, 15, 10, 5 and 3 points. The remaining points were used to validate the models. The point positions were determined on the basis of a soil brightness index map calculated from the UAS image, in order to distribute the points in areas of contrasted brightness. Root Mean Squared Error Prediction (RMSEP) obtained by cross-validation were 1.6 g.kg-1 and 28 g.kg-1 for organic carbon and clay respectively, with 20 points. Results showed ability to obtain acceptable precision (2 g.kg-1 and 48 g.kg-1) with only 3 points. This work was supported by the SolFIT research network of the BASC LabEx (Laboratory of Excellence) and by the TOSCA-PLEIADES-CO project of the French Space Agency (CNES).
Authors:
Jean-Marc Gilliot, Joël Michelin, Maxime Becu, Moustapha Cissé, Dalila Hadjar, Emmanuelle Vaudour
Institutions:
UMR ECOSYS, AgroParisTech, INRA, Université Paris-Saclay, DEFISOL